Leveraging AI in Business: From Competitiveness to Competitive Advantage - Part 2/4
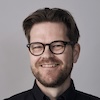

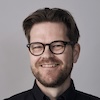
Leveraging AI in Business: From Competitiveness to Competitive Advantage - Part 2/4
This post continues the discussion on leveraging artificial intelligence (AI) to develop and enhance competitive advantage for businesses. My previous post dealt with maintaining competitiveness over the short term; this one extends the discourse into a long-term perspective. To keep this article from becoming unreadably lengthy, I will save the discussion on competitive advantage for my next piece.
Competitiveness in the Long-Term
The AI-based solutions described in my previous post are already available today and could well be in use within 1-2 years by all companies that wish to remain competitive on the international stage. Development will bring new features that will become widely used "standard solutions" for businesses. What these crucial solutions might be in the long term, no one can say with certainty, but I will next examine three themes around which very interesting developments are currently happening. These technologies are partly in existence today, and as they continue to evolve, it's easy to see their broad impact on businesses in the long run. These three themes are:
- Autonomous Agents
- IoT and Robotics
- Tailored Language Models
Autonomous Agents
Autonomous agents refer to software programs where an AI agent is given a goal, and the agent solves it completely independently without human intervention. Two AI-capabilities make this possible; reasoning and integration. The large language model's abilities for reasoning are used for creating the plans for achieving the specific goal, and for reflecting the results to control the quality of work. Integrations in turn provide autonomous agents abilities to connect and use external tools for achieving the goal.
In a simplified example the agent is assigned an objective which triggers following chain of events:
-
Assignment: The agent is given a goal, and it uses large language models to interpret the goal and its context. Example: A Digital Marketing Agent is given a goal of increasing client engagement and sales through targeted online campaign.
-
Planning: The agent plans its workflow, identifies necessary resources, and other agents that could be utilized in accomplishing the goal. Example: The Agent reasons the advertising strategy considering budgets, target groups and possible platforms (e.g. Google Ads, Facebook, LinkedIn), and creates a plan for implementation.
-
Execution: The agent operates independently and performs the tasks it has planned, by using e.g. internet search, external services and databases through API's and other agents. Example: The Agent sets up and launches the campaign, and utilizes ad platform API's to adjust bids, targeting and creatives.
-
Assessment: The agent assesses the actions and feedback it receives from internal and external systems. If necessary, it modifies its plans and methods for accomplishing the goal. The agent operates in an action-feedback-adaptation-loop until the goal has been reached. Example: The Agent analyzes incoming data from ad platforms and adjust strategy by reallocating budgets to better performing platforms and segments.
-
Completion: Finally, the agent delivers the finished solution to the user and uses the feedback received to improve its skills for next time. Example: The Agent compiles a comprehensive report with performance metrics, gained insights and recommendations and delivers the report to marketing team.
The marketing example above is simplified, but it illustrates how agentic frameworks can independently find ways to solve tasks, including defining the action plan and executing the actions. Until recently, executing such a programming task would have required at least human-defined automation workflow.
We are still in the early stages of Autonomous Agent framework development, but the interest and investments towards this technology is rapidly increasing. Major technology companies like Google and Microsoft have released their own solutions for implementing agents. There are also open-source code bases that allow for the construction of simple autonomous agents.
This architecture, utilizing agents in planning and executing actions, can also improve the performance of the language model used. For example, the performance of the older (and free) GPT-3.5 in certain tasks has been boosted to the level of the newer (and paid) GPT-4 by using an agent architecture. It seems teamwork is more efficient and creates better results also in digital agentic world, just like in real life.
For businesses, agents can offer a wide range of support in the future. Autonomous agents can handle and analyze large amounts of data more efficiently than humans, and they do not tire in monotonous or long-term tasks. They persist in finding ways to solve assigned tasks. In turn, humans can focus on more complex, strategic, and creative work, as well as interpersonal interactions that are still necessary for collaboration and development.
Ethan Mollick, a professor of Artificial Intelligence and Innovation at Wharton, has presented an interesting idea about how autonomous agents could be the key to broader utilization of AI in organizations. He notes that agents naturally fit into current business organizational structures and operating models. Instead of relying on employees to take over all AI-related tasks and utilize it, AI agents could act as individual consultants, employed in suitable tasks when needed.
IoT and Robotics
The Internet of Things (IoT) acts as a bridge between the digital and physical worlds through physical devices and sensors. While autonomous agents enable the automation of tasks and independent problem-solving in the digital world without human help, IoT combined with advancements in robotics offers similar possibilities in the physical world. This integration of IoT and robotics also has its own term: the Internet of Robotic Things (IoRT).
The development of robotic technology has significantly enhanced the physical capabilities of robots. AI, in turn, has added to the robots' arsenal autonomous, more precise, and faster learning, the ability to recognize and interpret the environment with new precision, and the ability to adapt their actions automatically in a changing environment and with new information. For example, Boston Dynamics' Atlas can solve ways to reach a desired destination by utilizing available materials like planks and scaffolding and perform complex physical actions like flips. Google DeepMind's robots have independently learned to play soccer, having been given only the objective to score goals.
Many tech giants are feverishly developing humanoid robots. For instance, Tesla has introduced plans to bring to market by 2025 an Optimus humanoid robot priced under $25,000, capable of performing diverse tasks and integrating into people's everyday lives. Figure AI, in collaboration with OpenAI, has introduced a robot that converses with the user using ChatGPT's voice features. With these robotics innovations, it is possible that in the future, robots will not only enhance the operation of industrial plants but also facilitate everyday chores, serve as assistants, and even colleagues in various work environments.
IoT data utilization and virtual technologies enable digital twin solutions, where a virtual version of a physical world object is created. These virtual models serve as powerful tools in testing, optimization, and decision support in controlled industrial environments. The same technology-enabled virtual training offers effective skill development in low-risk environments, enhancing workers' expertise and safety. Trainees can experience realistic situations, such as in an operating room, without the risks associated with actual training, reducing the need for physical resources, travel, and on-site training.
Tailored Language Models
Dozens of language models, the engines behind AI solutions, are currently available. Some are commercial, such as GPT-4, which underpins ChatGPT and Microsoft Copilot, while there are also several free open-source models like Llama3 and Mistral. Some are generic, all-encompassing, while others are narrower, focusing on specific themes. In addition to the language models themselves, the methods to supplement a language model with company-specific information are also continuously developing, offering more and more companies the opportunity to tailor their own corporate language model.
For example, McKinsey has already developed its own corporate language model, Lilli, which offers thousands of employees the opportunity to utilize the consulting firm's extensive knowledge archive. Similar examples can be found from various industries such as financial services, banking, healthcare, retail, and government. These industries benefit from tailored LLMs to improve:
- Content creation
- Personalized marketing
- Sentiment analysis
- Fraud detection
- Legal research
- Education
- Talent acquisition
- Customer service
- Policy analysis
Alongside tailor-made language models, companies can also utilize vertical AI solutions. Unlike horizontal AI, which aims for broad applications across various industries, vertical AI delves into the unique needs and challenges of a specific sector, such as healthcare, finance, or agriculture. In this case, a company does not need to tailor its own language model but can utilize a pre-implemented sector-specific solution.
These solutions integrate into industry operation models, and these solutions are developed using a deep understanding of the industry's unique challenges, processes, terminology, regulation, and business models. In industries where precision and regulation play a crucial role, such as healthcare and finance, the impact of vertical AI can be particularly significant as it ensures compliance and enhances decision-making. Such vertical language models can enhance the operations of the companies that utilize them to such an extent that operating competitively without them may be challenging.
One example of such development is Harvey, which is revolutionizing task management in law firms by automating routine tasks such as document review and case study. This frees legal professionals to focus on the more complex and strategic aspects of their work. Microsoft, for instance, offers industry-specific solutions to the healthcare sector.
Considerations for Decision-Makers
-
What tasks in your company could be delegated to autonomous agents? Could the time freed up for employees be used for more creative and strategic work, or better serving customers?
-
How could your company utilize IoT or robotics solutions? Could production, supply chain management, or customer experience be developed, for instance?
-
Are there vertical AI tools available for your industry? Does your company have valuable and useful information that would warrant considering customizing your own language model?
Finally
Predicting the long term is notoriously difficult, and with rapid development, it is practically impossible in the case of AI. The three themes presented in this post are currently developing very rapidly, and they are also partly interconnected. For example, agent architecture enables smarter collaboration of future robots, and tailored language models enable more companies to intelligently utilize IoT technologies tailored to their own businesses.
What new company-specific applications can be found for these and other AI-enabled solutions? Could this form a competitive edge for your company? From these starting points, I will continue the discussion in my next post.
How do you view the situation from your company's perspective? Please share your feedback and connect to discuss the topic further.